Be Your Own Prada:
Fashion Synthesis with Structural Coherence
Shizhan Zhu1,
Sanja Fidler2,3,
Raquel Urtasun2,3,4,
Dahua Lin1,
Chen Change Loy1
1Department of Informaiton Engineering, The Chinese University of Hong Kong
2University of Toronto
3Vector Institute
4Uber Advanced Technologies Group
[Full Paper]
[Codes and Dataset]
Abstract
We present a novel and effective approach for generating new clothing on a wearer through generative adversarial learning. Given an input image of a person and a sentence describing a different outfit, our model “redresses” the person as desired, while at the same time keeping the wearer and her/his pose unchanged. Generating new outfits with precise regions conforming to a language description while retaining wearer’s body structure is a new challenging task. Existing generative adversarial networks are not ideal in ensuring global coherence of structure given both the input photograph and language description as conditions. We address this challenge by decomposing the complex generative process into two conditional stages. In the first stage, we generate a plausible semantic segmentation map that obeys the wearer’s pose as a latent spatial arrangement. An effective spatial constraint is formulated to guide the generation of this semantic segmentation map. In the second stage, a generative model with a newly proposed compositional mapping layer is used to render the final image with precise regions and textures conditioned on this map. We extended the DeepFashion dataset by collecting sentence descriptions for 79K images. We demonstrate the effectiveness of our approach through both quantitative and qualitative evaluations. A user study is also conducted.
Fashion GAN
We decompose the overall generative process into two relatively easier stages, namely the human segmentation (shape) generation (corresponding to the desired/target outfit) and texture rendering.
In our first stage, we first generate a human segmentation map by taking the original segmentation map and the design coding into account, to serve as the spatial constraint to ensure structural coherence.
In the second stage, we use the generated segmentation map produced by the first generator, as well as the design coding, to render the garments for redressing the wearer.
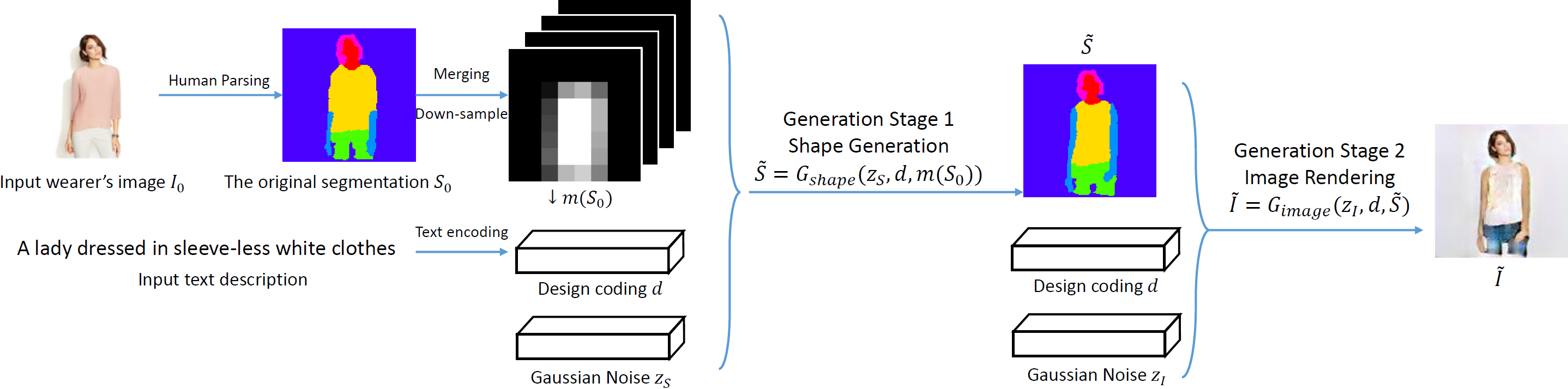
Qualitative Results
1. Each row is generated from the same person while each collumn is generated from the same description.
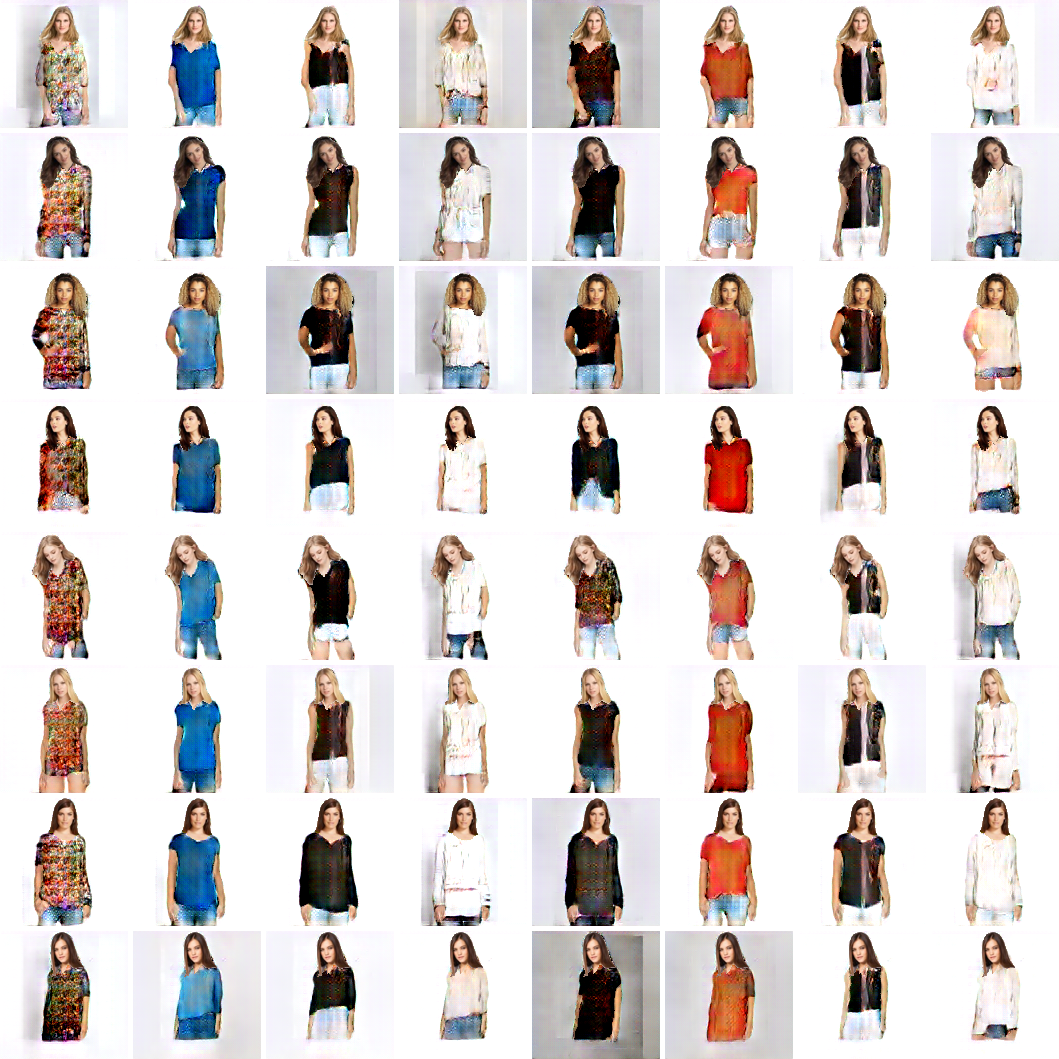
2. Each row is generated from the same person as well as the same description.
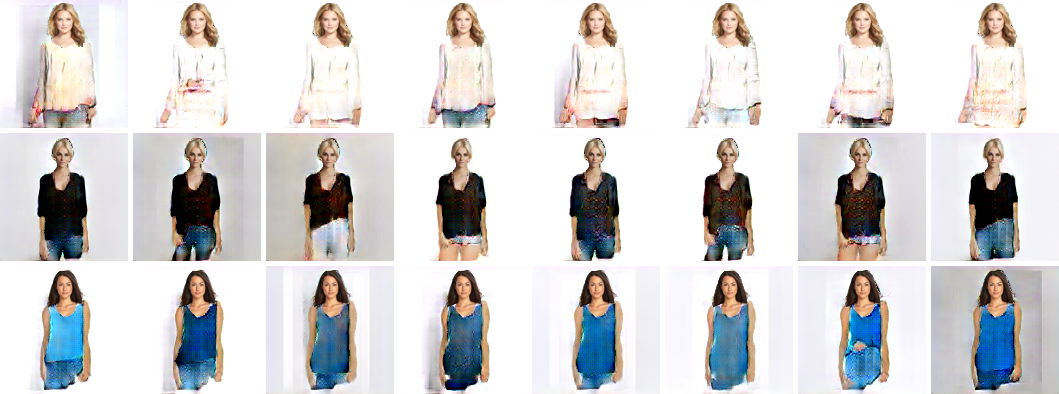
3. Interpolation along the way. Row 1: Interpolation along the shape as well as the texture. Row 2: Only along the texture with the shape fixed. Row 3: Only along the shape with the texture fixed.
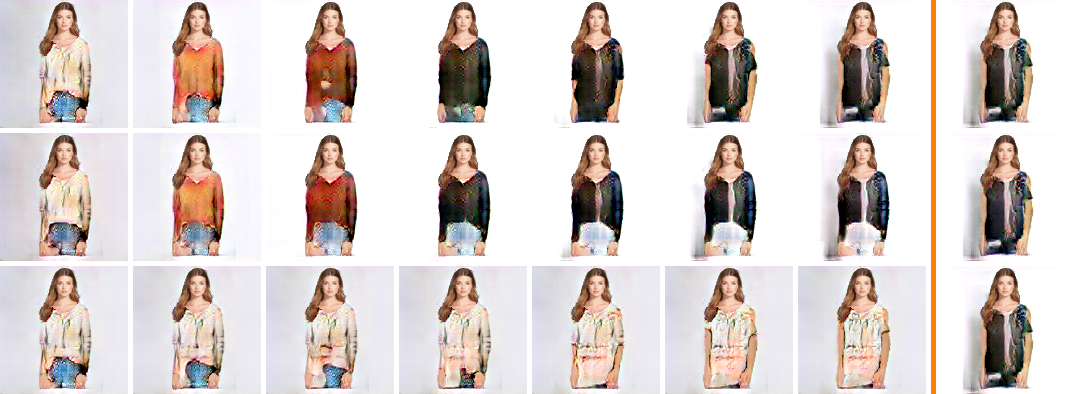
Citation
@inproceedings{zhu2017be,
author = {Shizhan Zhu, Sanja Fidler, Raquel Urtasun, Dahua Lin, Chen Change Loy},
title = {Be Your Own Prada: Fashion Synthesis with Structural Coherence},
booktitle = {International Conference on Computer Vision (ICCV)},
year = {2017}}