Crafting a Toolchain for Image Restoration by
Deep Reinforcement Learning
2 SenseTime Research 3 Sun Yat-sen University
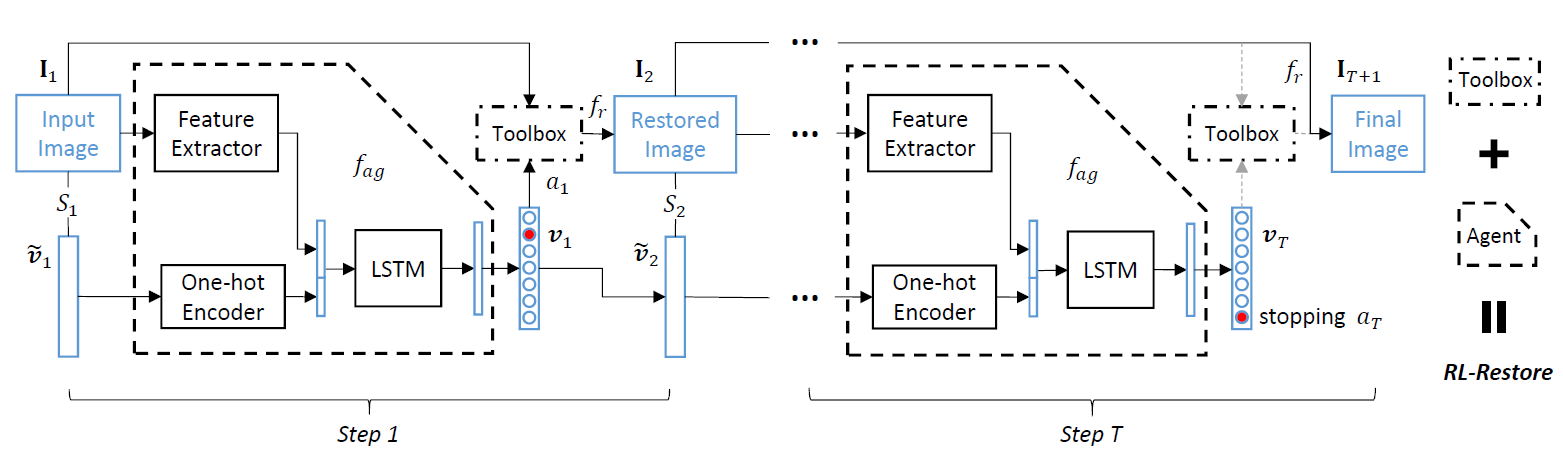

Abstract
We investigate a novel approach for image restoration by reinforcement learning. Unlike existing studies that mostly train a single large network for a specialized task, we prepare a toolbox consisting of small-scale convolutional networks of different complexities and specialized in different tasks. Our method, RL-Restore, then learns a policy to select appropriate tools from the toolbox to progressively restore the quality of a corrupted image. We formulate a step-wise reward function proportional to how well the image is restored at each step to learn the action policy. We also devise a joint learning scheme to train the agent and tools for better performance in handling uncertainty. In comparison to conventional human-designed networks, RL-Restore is capable of restoring images corrupted with complex and unknown distortions in a more parameter-efficient manner using the dynamically formed toolchain.
Materials
Code and data
Citation
@inproceedings{yu2018crafting, author = {Ke Yu, Chao Dong, Liang Lin, and Chen Change Loy}, title = {Crafting a Toolchain for Image Restoration by Deep Reinforcement Learning}, booktitle = {Proceedings of IEEE Conference on Computer Vision and Pattern Recognition (CVPR)}, month = {June}, year = {2018} }