Chen Huang1,2, Chen Change Loy1,3, and Xiaoou Tang1,3
1Department of Informaiton Engineering, The Chinese University of Hong Kong, 2SenseTime Group Limited,
3Shenzhen Institutes of Advanced Technology, Chinese Academy of Sciences.
IEEE Conference on Computer Vision and Pattern Recognition (CVPR) 2016, Las Vegas, USA
[PDF]
[Supplementary]
[Poster]
[Code]
Abstract
Attributes offer useful mid-level features to interpret visual data. While most attribute learning methods are supervised by costly human-generated labels, we introduce a simple yet powerful unsupervised approach to learn and predict visual attributes directly from data. Given a large unlabeled image collection as input, we train deep Convolutional Neural Networks (CNNs) to output a set of discriminative, binary attributes often with semantic meanings. Specifically, we first train a CNN coupled with unsupervised discriminative clustering, and then use the cluster membership as a soft supervision to discover shared attributes from the clusters while maximizing their separability. The learned attributes are shown to be capable of encoding rich imagery properties from both natural images and contour patches. The visual representations learned in this way are also transferrable to other tasks such as object detection. We show other convincing results on the related tasks of image retrieval and classification, and contour detection.
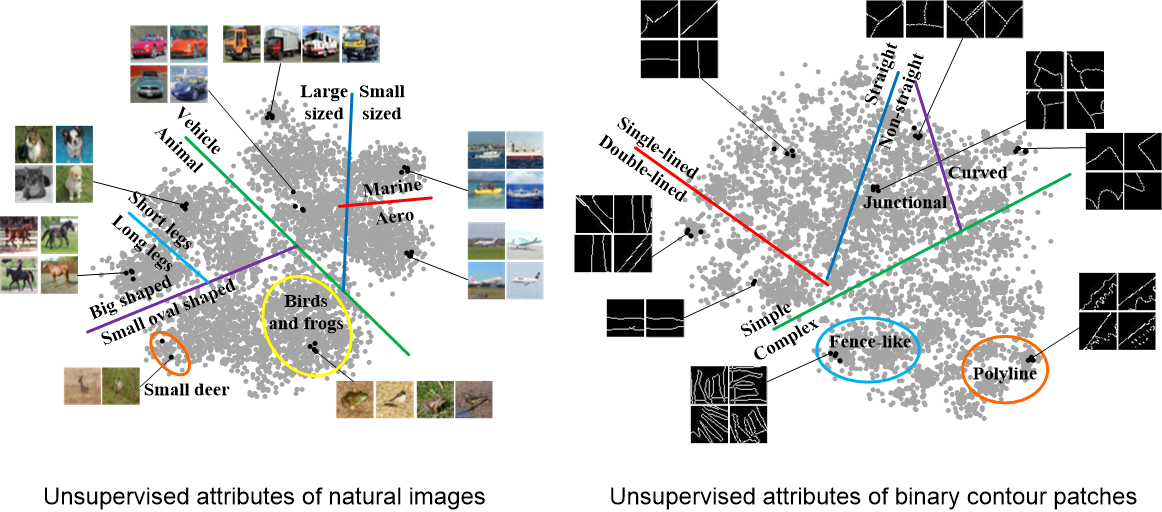
Method and applications
Pretraining from scratch with modified unsupervised discriminative clustering + finetuning with weakly-supervised hashing
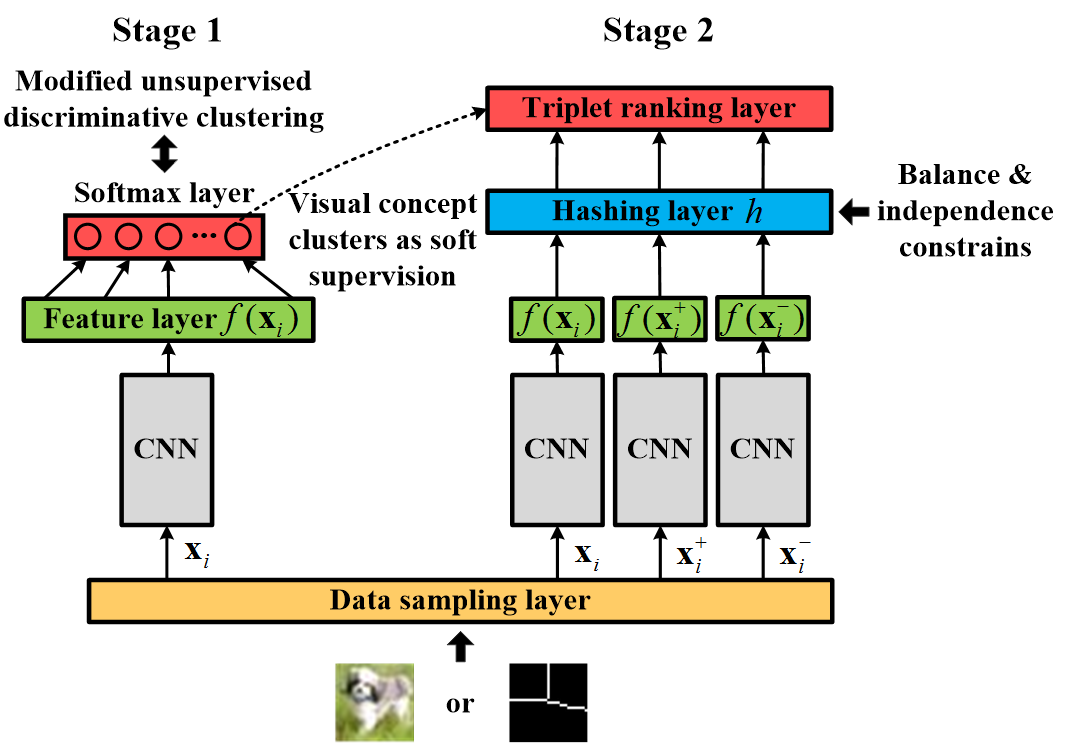
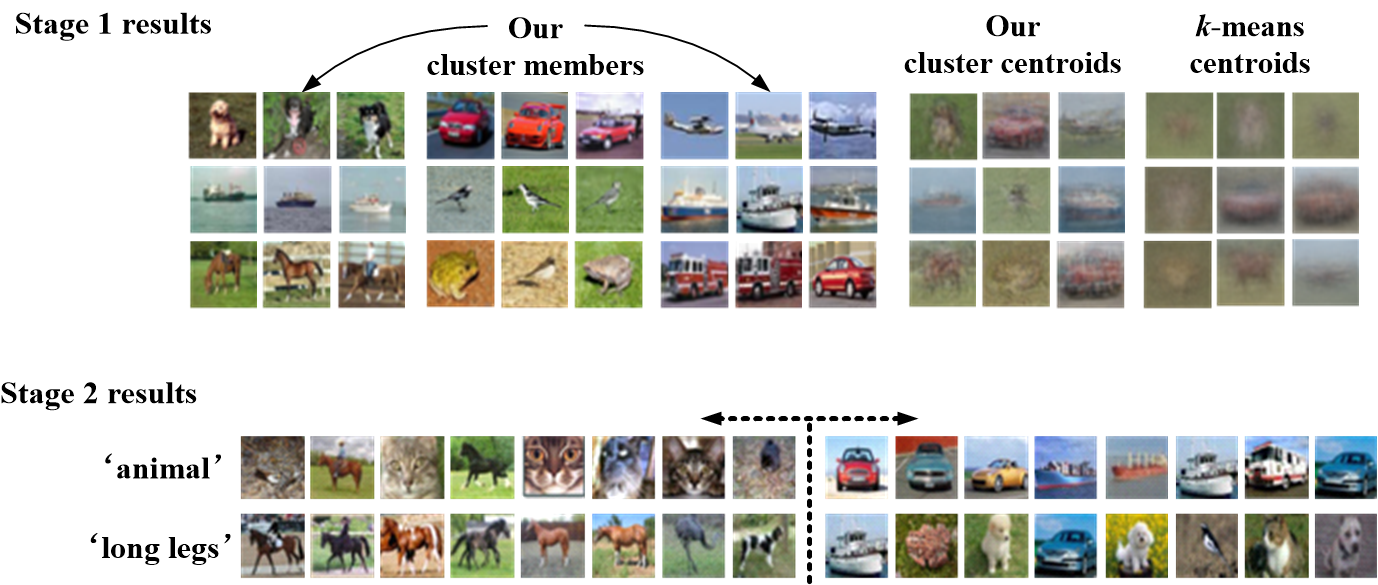
Enable diverse vision applications:
- Unsupervised pre-training for object detection
- Unsupervised learning for image retrieval
- Unsupervised learning for image classification
- Unsupervised learning for contour detection